AI Is Making Data Security More Challenging
The latest wave of generative AI advancements unlocks new levels of efficiency, productivity, and insights — yet these outcomes come at a cost, specifically to data security.
It bears repeating: 8 out of 10 respondents agree that AI is making data security more difficult.
When users feed data into public LLMs, it leaves their environment, and subsequently, their control. Many AI models use new information that’s inputted as source material. This presents a higher risk of attack and other cascading security threats.
Making matters more complex, most models are black box-oriented, so understanding the security impact of specific data lapses is incredibly difficult.
“These models are very expensive to train and maintain and do forensic analysis on, so they carry a lot of uncertainty. We’re not sure of their impact or the scope.”
Joe Regensburger
VP Research at Immuta
Rapid Adoption & Growing Pains
Exacerbating the security risks even more is the rapid pace at which companies are adopting AI to keep up with the competition and market. More than half of respondents (54%) say their organization already leverages at least four AI systems or applications.
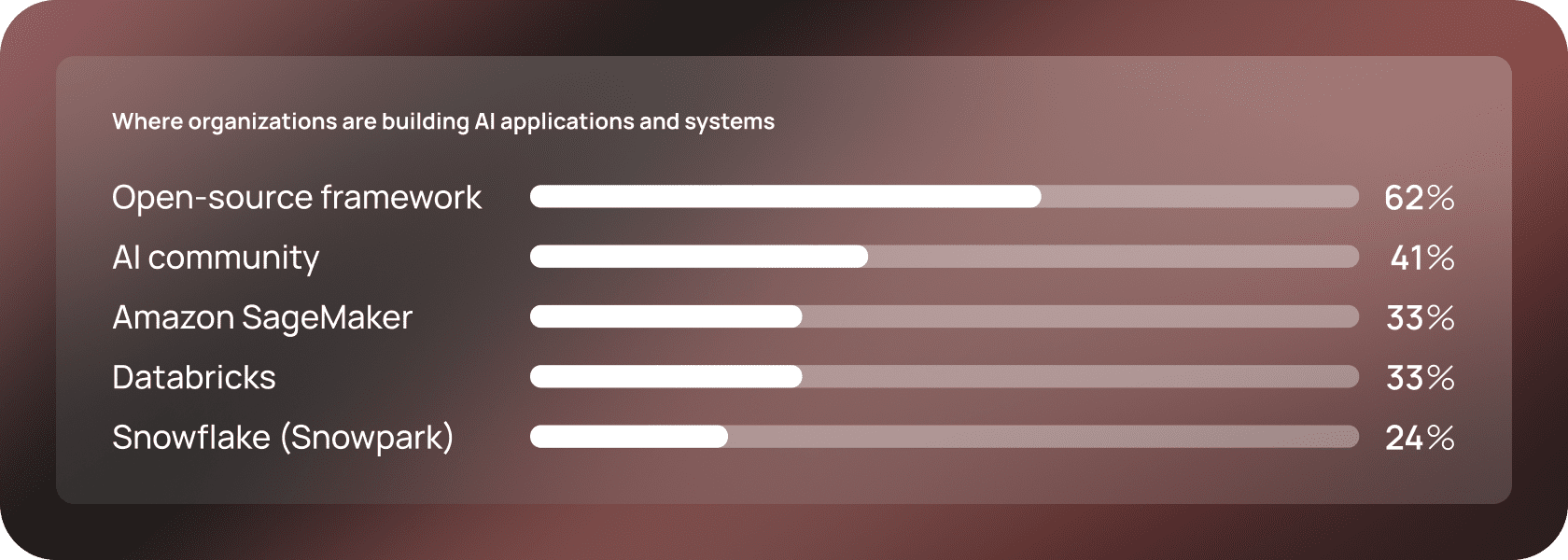
The result of such fast-paced adoption is simple. Some companies are building homegrown solutions and smaller, proprietary LMs, though not as frequently. More commonly, the high cost of training and maintaining LLMs means most organizations are sending their data off to a third party, amortizing the cost across a large number of people by using a commercial model like OpenAI or Gemini.
However, the potential security impact of these models is much greater. Organizations don’t have an established relationship or degree of trust with these vendors, and they don’t know how the third parties will use their data. It’s far too easy to enter a prompt that includes sensitive data into ChatGPT’s open interface, even while AI remains largely unregulated.
All of this adds up to massive uncertainty. True AI security means answering questions like:
- Who’s using your data?
- Where is your data going?
- What is your data being used for after it leaves your environment?
Closer to home, incorporating AI into existing tech is its own challenge. Nearly half of data leaders (46%) rate the difficulty of integrating AI with legacy technologies as difficult or extremely difficult. Only 19% think it’s relatively easy.
The prompt engineering phase is vital for easing this transition. As more traditional databases start to implement a vector database infrastructure, users will be able to leverage unstructured text to search more efficiently. This will allow them to provide support materials specific to their organization, unlocking the niche knowledge models needed for better output.
“To reduce hallucinations, it’s important to provide sufficient domain-specific context to each prompt.”
Joe Regensburger
VP Research at Immuta
Integrating AI with legacy technologies brings its share of challenges. First and foremost is security: 27% of respondents point to data security concerns as the biggest challenge when integrating AI with legacy software. They also cite major integration challenges like employee skills gaps (24%), data quality and accessibility issues (15%), and ethical and regulatory compliance concerns (11%).
Threats Posed by AI
The number-one data security threat with AI and LLMs is inadvertent exposure of sensitive information by LLMs, with 55% of respondents agreeing it’s a significant risk. And at least half of data leaders are concerned about other key data security threats:
- Adversarial attacks by malicious actors via AI models (52%)
- Inadvertent exposure of sensitive info to LLMs via user prompts (52%)
- Use of purpose-built models for unauthorized tasks (52%)
- Shadow AI, or using AI in unsanctioned ways outside of IT governance (50%)
While the exposure of sensitive information by LLMs is a top-perceived threat, respondents rank adversarial attacks via AI models as a slightly bigger security threat overall. And attacks enabled by AI are also on the rise: Over half of respondents (57%) say that they’ve seen a significant uptick in AI-powered attacks in the past year.
While 53% of organizations acknowledge cybersecurity as a generative AI-related risk, according to McKinsey just 38% are working to mitigate that risk.
The negative outcomes from these attacks are steep. They range from exposure of sensitive data (48%) and proprietary information or IP (38%), to performance disruptions (40%) and system downtime (36%). Another 26% had to pay regulatory fines or penalties.
As much innovation and potential as AI offers, the technology can — and will continue to be — used for nefarious purposes. The effects of those attacks, in reputational damage, operational impacts, and financial penalties, to name a few, are a harsh reminder of AI’s shadow side.
Do the Right Thing With Ethical AI
Despite unfolding guidelines like the US AI Bill of Rights and the EU AI Act, AI regulation remains in its infancy. However, organizations still have a massive responsibility to define and uphold the ethics of their AI usage. This ranges from maintaining human oversight and remaining upfront about how LLMs are trained, to protecting users’ sensitive data from exposure and leaks.
What is on data leaders’ minds when it comes to AI ethics? Close to half of respondents (48%) cite security as the most pressing ethical concern with AI. Other pressing ethical issues include:
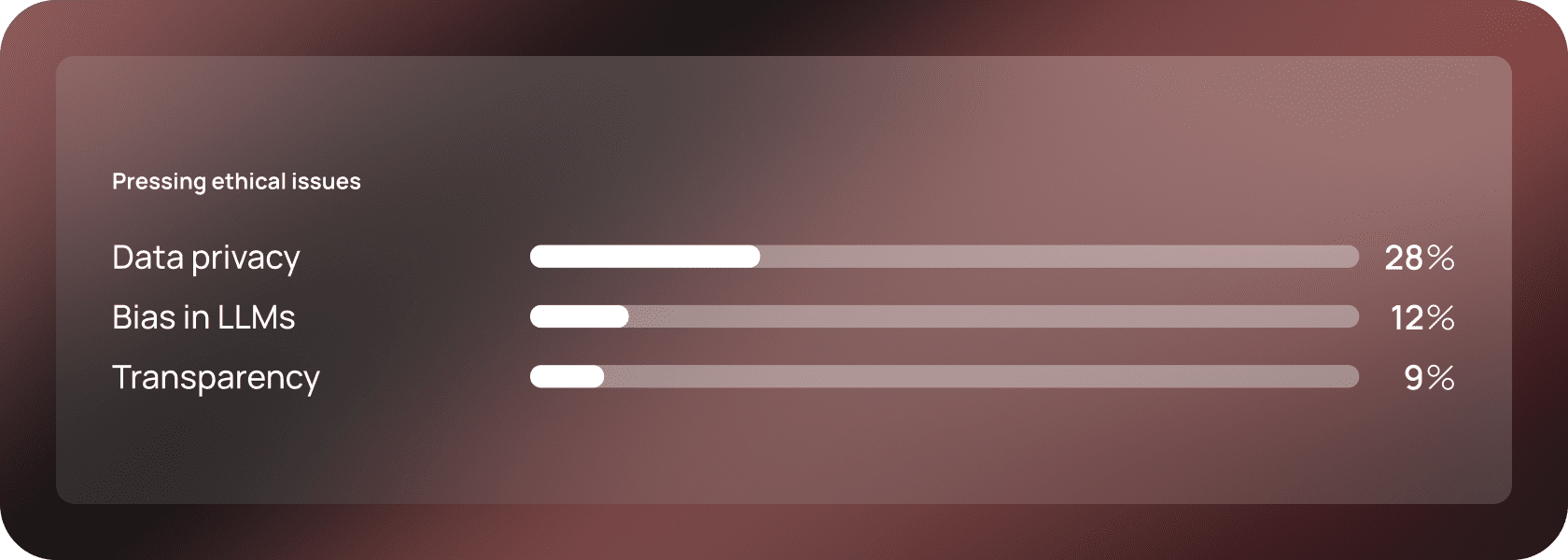
Although only 12% of respondents rank bias as their most pressing ethical concern, data leaders across the board are thinking about it often. Around two-thirds (65%) say that AI bias is a moderate concern for them.
The security impacts of bias can be far-reaching. For instance, bias leaves organizations vulnerable when a model has a limited threat detection scope or overlooks risks — and the potential fallout is too great to ignore when data protection is at stake.